While “Big data” has garnered a lot of attention over the last number of years, many managers struggle in deciding where to begin. They can often be mistakenly seduced by technology companies with the promise of an IT solution to the (big) data problem. By first distinguishing between the two different ways that data can be leveraged, this article suggests a route to navigate the terrain. It introduces the QuDa model as the foundation from which a (big) data initiative can be mapped. Its fundamental premise is that it is managers not technology that give meaning to data.
One of the questions that I frequently encounter in my work with executives concerning so called “big data” is where to start. Unfortunately, many look to their IT organisation for guidance, seeing the challenges as being technical in origin and consequently having an IT solution. They can often be convinced – wrongly in my opinion – of the need to buy technologies like a data warehouse, analytical tools, or perhaps event to invest in Hadoop. While all these technologies might help, my advice is to first get a handle on the data your organisation has, its quality and how you currently use it. With this understanding, you can then start to become more sophisticated in thinking about how you might use data and the outcomes being sought. Of course, it is crucial to first acknowledge what you are trying to achieve. This is why it is important at the outset to recognise the distinction between the exploration of data and the exploitation of data; this helps in establishing a focus for any initiative. With this understanding, particularly how the two concepts interrelate, managers can then begin to map out what they are seeking to achieve. In this article I argue that there are four possible outcomes when exploring data and that these outcomes can help in providing the clarity that is all too often absent.
Exploration and exploitation
Given all the attention that “big data” has received over the last few years, managers can be forgiven for thinking that this is something new. It is not. The fact is managers and their organisations have always struggled to control and leverage data. This is why computers were introduced into organisations in the first place. The paradox is that the technologies that were supposed to help manage data are now causing a data deluge. The ‘big’ really just signifies that there is a lot more of data around today! Distinguishing between exploration and exploitation can be very useful in thinking about data and how it might be leveraged. It also helps in differentiating between using analytical and business intelligence (BI) tools and other applications of IT, such as an ERP system or an IT-system for online ordering. Actually, the concepts can be considered as two sides of the same coin, but more about this later.
Exploration is using data to better understand something; whether this is about your customers, your operations, your products, your supply network, the marketplace, etc. This data may be internally generated from operations, from devices, machines or assets, or result from direct interactions with customers and ecosystem partners. Sometimes it can come from external sources, such as comments about your products or services posted on Facebook or tweets on Twitter. This challenge is to make sense of this data. For an electricity provider, for example, this might be converting 200 million meter readings per annum to make sense of the power consumption of customers in order to optimise the production of electricity. Insurance companies might want to know the likelihood that a claim is fraudulent. While often referred to as “insight” by marketers, these examples are essentially about generating understanding and discovering new knowledge. Some of the ways that data is being explored include: modeling risk, conducting customer churn analysis, predicting customer preferences, targeting ads, and detecting threats and fraudulent activity. This often requires that datasets from diverse sources be combined and examined. For example, Her Majesty’s Revenue and Customs (HMRC) is combining external data from credit and other institutions with its own internal data to identify potential under-declaration of tax liabilities.
The exploration of data is also revealing some unexpected results that themselves required some creative thinking. Public health researchers, for example, have found a spike in Google search requests for terms like “flu symptoms” and “flu treatments” a few weeks before there is an actual increase in flu patients coming to hospital emergency rooms. Central banks are currently studying whether keyword searches, reported by Google as soon as the queries take place, can provide lead indicators of consumer demand before official statistics become available.
Sometimes a company can be pro-active in gathering data. For example, many have implemented customer relationship management (CRM) technologies to glean information about customers (demographic details, choices and preferences) and information from customers (as a result of their interactions and exchanges). One of the things they then look to do is use this information to tailor offerings to customers based on anticipated needs that they glean from historical data. Amazon, for example, claim that 30% of its sales are generated as a result of its recommendation engine: “customers who bought this book also purchased….”
Initiatives to explore big data are getting ever more sophisticated. Using video, some retailers are now building systems to better understand customer behaviour when they are in the store. In most cases, this video data is taken directly from a store’s existing security cameras. That feed is analysed and correlated with sales data. Some retailers are also integrating with data from hardware such as radio-frequency identification (RFID) tags and motion sensors to track how often a brand of cereal is picked up or how many customers turn left when they enter a store. Using video, luxury retailer Montblanc generates maps showing which parts of the store are most-trafficked and use this knowledge to decide where to place in-store decorations, salespeople, and merchandise. Such analysis can often throw up insights that refute conventional wisdom. For example, many food manufacturers pay a premium for their products to be displayed at the end of an aisle. But what retailers are finding is that customers actually pay greater attention to products placed in the centre of an aisle.
Exploitation is using data to take advantage of information asymmetries; it is essentially about making the invisible visible. These asymmetries arise when one party to a transaction or interaction or potential transaction/interaction has more or better information than the other. This usually results in costs which are often referred to as “transaction costs.” Large vertically integrated companies were established to reduce transaction costs in open markets. Today, the use of technology can greatly reduce transaction costs both in markets and within organisations. Thus, by identifying these asymmetries that are leading to transaction costs, an organisation can avail of opportunities, through the medium of technology, to potentially change what the organisation does, even providing a source of competitive differentiation.
For example, manufacturers often hold stocks of raw materials to buffer against uncertainty in their supply chains – what is often referred to as “safety stock.” This uncertainty is caused by having an incomplete picture of production processes, schedules and stock levels at suppliers. Making this information available to the manufacturer reduces uncertainties caused by these information asymmetries. Armed with this information, manufacturers no longer need to tie up working capital in raw material inventories and risk obsolesces and can execute a JustIn-Time (JIT) strategy. JIT is essentially about replacing inventory with information. It is information asymmetries that have seen the rise of price comparison websites where shoppers visiting a store can use their mobile phones to scan bar codes to see if there is a better deal available elsewhere.
Being ambidextrous: leveraging exploration and exploitation. Many of the examples of big data use actually rest on the interplay between exploration and exploitation. Thus, by generating new insights from exploring data, an organisation may choose to then exploit any information asymmetries that may be revealed. For example, insights from historical tax returns data and other external data (exploration) are enabling HMRC to identify factors that indicate potentially under declaration of tax liabilities. Using this knowledge it has built an (exploitation) application that automatically checks all tax returns submitted by citizens against this ‘signature’, flagging up those that are potentially fraudulent for further scrutiny. The recommendation engine of online retailer Amazon (‘customers who bought this book also….’) is based on the same ambidextrous principle, where exploration of book purchases across all its customers has enabled the company to generate new knowledge about customers and exploit this insight and build an application to make purchasing suggestions for customers.
Exploring data to discover new knowledge: The QuDa Model
The historical and dominant application of IT is in organisations and their processes and across their ecosystem is to exploit information asymmetries. How this is done is well established, but unfortunately most organisations still struggle to achieve expected benefits. To capture the exploration of big data and the use of analytical tools to generate new knowledge and insight, a good starting point is to borrow from Donald Rumsfeld’s words at his infamous 2003 press conference: “There are known knowns. These are things we know that we know. There are known unknowns. That is to say, there are things that we know we don't know. But there are also unknown unknowns. There are things we don't know we don't know.” These statements can be used to create a model to capture the different possible outcomes from exploring data.
These four outcomes can be positioned on a framework that maps the question against the data. The reason for the “question” dimension is that knowledge emerges from answering posed questions. Einstein captured the importance of questioning and getting the question right when he noted: “If I had an hour to solve a problem and my life depended on the solution, I would spend the first fifty-five minutes determining the proper question to ask, for once I know the proper question, I could solve the problem in less than five minutes.” The data dimension signifies the potential source for uncovering this knowledge.
Defined question/known data. This is where the question being answered is already known and the data to help in answering the question has already been pre-determined. These are the known knowns – things that we know we know. Good examples here are reports and dashboards that contain information that answers pre-established questions. Sales reports typically answer questions about sales by region, sales person, product line, etc. The knowledge being sought is determined by the question; to answer the question requires particular data. Reports and dashboards actually provide the answers pre-determined questions. Financial reports, sales reports, reports showing actual key performance indicators (KPIs) against planned are examples of such reports.
Known data/undefined question. This situation exists where there is data in the organisation that might reveal something about customers, operations, suppliers, products, etc. but the question to uncover this knowledge has not yet been posed. GE, for example, collects terabytes of telemetry data on the performance of its engines. The company knows that there is knowledge in this data that has not yet been revealed about these engines. The challenge is to construct an inquiry to uncover this knowledge as these are the unknown knowns – what, as an organisation, we don’t know we know.
Defined question/un-known data. In this scenario we have a question – there is something that we wish to know. However, the data to provide an answer to this question has not yet been identified or precisely defined; this is the known unknown – what we know is that we as yet don’t know the answer to a particular question. Often getting this knowledge will demand refining the initial question. Instrumenting products with sensors, for example, provides opportunities to capture data on a “product in use.”
Undefined question/un-known data. This situation acknowledges that there is new knowledge to be discovered but that we have yet to either pose the question or identify the relevant data. These are the unknown unknowns. For example, Google announced some time ago that it is collecting as much data as it can even though at this stage it doesn’t know how it is going to use it or what it might tell them.
Framing questions can unlock innovation
The QuDa model emphasises two important aspects of exploring data to discover new knowledge. First, it emphasises the importance of precisely framing the question in the process of generating insight. Second, it points to the criticality of accurately identifying and selecting the data where the answer to the question lies.
The framing of a question n is, in essence, how it is posed. So, for example, if somebody was trying to solve a mathematical problem and wanted to seek your help there are several ways they could ask. Posing a question like: “Can you help me with my mathematics?” is less precise than “What is x when x^2 + 2x - 24 = 0?”
Both of these questions are asking for help but one is framed in a better way to get a more accurate and precise answer. So you might be able to respond positively to the first question but be unable to solve the mathematical expression in the second which is really where help was needed.
With a well-defined question, where the data to generate an answer is known or can be determined, the question can be considered as structured – the ‘known known’s’. Standard reports typically provide answers to these questions.
Examples include:
- What are the factors that indicate a customer is likely to defect?
- Given current customer profiles, to which customers should we mail or new catalogue?
- Which products did our customers purchase most frequently last month?
- How much inventory do we have a product X?
- What were sales last week in the Southwest region?
Questions posed of data are often characterised in four ways:
- What happened (WHAT descriptive)
- Why it happened (WHY diagnostic)
- What will happen (WHEN predictive)
- How I can make it happen (HOW TO prescriptive)
However, questions are not always framed in a way to generate an unbiased, genuine answer. Some ways of framing questions can be designed in a way to get an answer that the person wants. Framing a question in a particular way can distort, twist, and influence the answer, defeating the point of asking the question in the first place. A question should be framed to elicit a genuine, unbiased answer. Frequently, political polls have framed questions to get particular answers to questions (and poll results).For instance take the questions:
“Who do you want Trump or Clinton to be America's next President?”
“Do you want someone with more business experience or less experience to be the next president?”
These are two questions about the same topic but framed differently. The first one is more fair and balanced. The second question is phrased or slanted in such a way to get the person to support Trump.
Asking the right question is only part of what is required. The question has to be answered; this is achieved through a process of knowledge discovery by interrogating the data, often by identifying additional data that may not be readily available. This is essentially a cognitive process that takes place in the minds of managers. As it typically takes place in teams, it has also a strong social element. This process of sense making is a voyage of discovery. It is important to never lose sight of that fact that it is people (i.e. managers and data scientists) not the technology that give meaning to data and make sense of it. Technologies, like analytical tools, merely augment human cognitive processes.
Sometimes, instead of a posing a direct question, some hypothesis is established with the data used to explore it further. A hypothesis is essentially a proposed explanation for some phenomenon. For example, that customers living in a particular part of the city buy a certain product. This hypothesis is then tested using data and evaluated as to whether it is proven to be ‘true’ or ‘false.’
It is perfectly valid for a hypothesis to start out as a hunch or ‘gut feeling’; this is where experience can come into play. However, we also need to be aware of biases and assumptions that we hold. For example, the confirmatory bias relates to the fact that we only ‘see’ data that confirms a particular point of view. We also need to be aware of spurious correlations is it does not indicate causation: what appear to be relationships between things can be just random.
Mapping a big data initiative
The QuDa model can also be used to guide a big data or analytics initiative. Much of the writing on big data presents examples of companies combining data sets, doing predictive analytics, etc. While useful in getting executive attention, it can all seem very daunting for a manager with little experience. Success with any big data initiatives demands first understanding data: data you have and don’t have; the role data plays in your existing business model; and thinking about how you might use data and the outcomes we are looking to achieve.
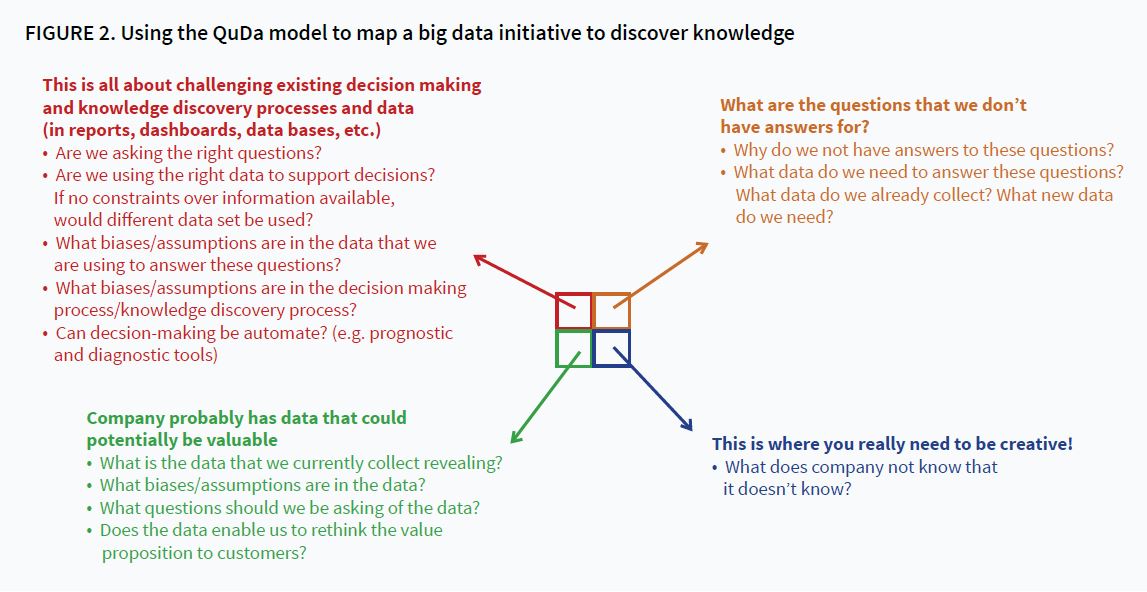
My advice is to start in the top left hand quadrant with the “known knowns.” This essentially demands challenging existing way of making decisions and the data used in making these decisions. Look at the BI reports and dashboards that managers use (or at least get or have access to – many are often not consulted or read). Most were probably been designed some years ago and may not be fit for purpose today. The constraints of data available at the time they were developed will also likely have influenced what is presented. Today richer and more comprehensive data may be available, but managers can continue to use these old reports. The layout and data on reports and dash boards is also likely to have been influenced by the particular biases and assumptions of the person who designed the report. Perhaps assumptions, valid when the report was initially designed, are now no longer appropriate in today’s environment. Furthermore, what is the quality of the data underpinning the report like?
Once this has been completed, existing data can be explored to assess whether or not it may be able to reveal new insights or knowledge – the “unknown knowns.” What questions can be posed of this data? Of course, we need to know what data we already collect and generate. This is not a trivial exercise; while most organisations are awash with data, discovering this and where it is located can be a challenge. This data might reveal insights that enable us to predict future states or behaviour if only it could be harnessed.
We can then move to the top right-hand quadrant. This is where we have questions but have not determined the data that will help in revealing answers to these questions – the “known unknowns”. Why do we not have answers to these questions is often worth exploring. Sometimes the question can be too loosely defined, making it hard to determine the data that might potentially provide an answer. It requires continual refinement in order to be more precise. Achieving this end is usually an iterative process as through a learning process sense is made of data. Examples of unstructured questions include:
- What is the demographic and psychological makeup of our potential high-value customers?
- Which hurts the bottom line more: inventory holding costs or hiring staff to handle frequent deliveries?
- How effective was our last marketing campaign?
- How do our customers migrate between segments?
The bottom right quadrant is really where you can start to be provocative: what does the organisation not know that it doesn’t know!
The figure presents some guidance for executing in each of the four quadrants of the QuDa model.
Conclusions
Managers should not be daunted by big data. My advice is that they should forget about the “big” label and just concentrate on data. Be clear as to whether what is being sought is the exploration or exploitation of data. The QuDa model presented in this article is to help managers first figure out the possible outcomes from an initiative what they are looking to achieve. It forces them to explore the data they have and don’t have as well as providing them with an understanding as to the process through which new insight will be gleaned. Mapping your exploration initiative will always lead you to return to the top left hand quadrant, for example, the “known unknowns” eventually become the “known knowns.” But it should also highlight that it is a never ending journey; a continual process of inquiry, learning and discovery as you move in and out of the four quadrants.
The article was published in European Business Review May - June 2016.